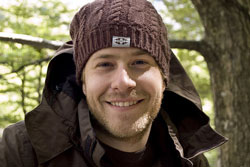
Nicholas Carlevaris-Bianco
nickcarlevaris@gmail.com
Google Scholar
Publications
My research focuses on perception for mobile robotics, using tools from computer vision, machine learning, and statistical signal processing for map building, navigation and scene understanding. I am specifically interested in developing algorithms that are robust to dynamic changes in the environment, with the end goal of helping to enable long term autonomy. I was a PhD Candidate in Electrical Engineering: Systems at the University of Michigan and a Graduate Student Research Assistant in the Perceptual Robotics Laboratory.
Journal
University of Michigan North Campus Long-Term Vision and Lidar DatasetNicholas Carlevaris-Bianco, Arash K. Ushani, and Ryan M. Eustice,
International Journal of Robotics Research, 2016. (In Press). [PDF] [BibTeX]
Long-Term Mapping Techniques for Ship Hull Inspection and Surveillance Using an Autonomous Underwater Vehicle
Paul Ozog, Nicholas Carlevaris-Bianco, Ayoung Kim and Ryan M. Eustice,
Journal of Field Robotics, Special Issue on Safety, Security and Rescue Robotics, 2015. [PDF] [BibTeX]
Generic Node Removal for Factor-Graph SLAM
Nicholas Carlevaris-Bianco, Michael Kaess, and Ryan M. Eustice,
IEEE Transactions on Robotics, 2014. [PDF] [BibTeX]
Conference and Workshop
Continuous-Time Estimation for Dynamic Obstacle TrackingArash K. Ushani, Nicholas Carlevaris-Bianco, Alexander G. Cunningham, Enric Galceran and Ryan M. Eustice,
In Proceedings of the IEEE/RSJ International Conference on Intelligent Robots and Systems, Hamburg, Germany, September 2015. [PDF] [BibTeX]
Learning Visual Feature Descriptors for Dynamic Lighting Conditions
Nicholas Carlevaris-Bianco and Ryan M. Eustice,
In Proceedings of the IEEE/RSJ International Conference on Intelligent Robots and Systems, Chicago, September 2014. [PDF] [BibTeX]
Conservative Edge Sparsiļ¬cation for Graph SLAM Node Removal
Nicholas Carlevaris-Bianco and Ryan M. Eustice,
In Proceedings of the IEEE International Conference on Robotics and Automation, Hong Kong, June 2014. [PDF] [BibTeX]
Long-Term Simultaneous Localization and Mapping with Generic Linear Constraint Node Removal
Nicholas Carlevaris-Bianco and Ryan M. Eustice,
In Proceedings of the IEEE/RSJ International Conference on Intelligent Robots and Systems, Tokyo, Japan, November 2013. [PDF] [BibTeX]
Generic factor-based node marginalization and edge sparsification for pose-graph SLAM
Nicholas Carlevaris-Bianco and Ryan M. Eustice,
In Proceedings of the IEEE International Conference on Robotics and Automation, Karlsruhe, Germany, May 2013. [PDF] [BibTeX]
Learning temporal co-observability relationships for lifelong robotic mapping
Nicholas Carlevaris-Bianco and Ryan M. Eustice,
IROS Workshop on Lifelong Learning for Mobile Robotics Applications, Vilamoura, Portugal, October 2012. [PDF] [BibTeX]
Visual localization in fused image and laser range data
Nicholas Carlevaris-Bianco, Anush Mohan, James R. McBride and Ryan M. Eustice,
In Proceedings of the IEEE/RSJ International Conference on Intelligent Robots and Systems, San Francisco, CA, September 2011. [PDF] [BibTeX]
Multi-view registration for feature-poor underwater imagery
Nicholas Carlevaris-Bianco and Ryan M. Eustice,
In Proceedings of the IEEE International Conference on Robotics and Automation, Shanghai, China, May 2011. [PDF] [BibTeX]
Initial results in underwater single image dehazing
Nicholas Carlevaris-Bianco, Anush Mohan and Ryan M. Eustice,
In Proceedings of the IEEE/MTS OCEANS Conference and Exhibition, Seattle, WA, September 2010. [PDF] [BibTeX]
Thesis
Long-Term Simultaneous Localization and Mapping in Dynamic EnvironmentsNicholas Carlevaris-Bianco
PhD thesis, Department of Electrical Engineering and Computer Science, University of Michigan, Ann Arbor, MI, January 2015. [PDF] [BibTeX]
Code & Data
Generic Linear Constraint Node Removal
The generic linear constraint (GLC) framework provides a method to remove nodes from SLAM graphs. This can be used for graph maintenance and to reduce computational complexity during long term SLAM. GLC produces a new set of factors over the elimination clique given only the existing factors as input. GLC works in graphs with less-than-full DOF constraints (e.g., bearing-only, range-only.), and avoids inconsistency found in methods based on measurement composition.My GLC node removal code is implemented and available for download within Michael Kaess's iSAM repository available at: http://people.csail.mit.edu/kaess/isam/
Please email me (nickcarlevaris@gmail.edu) with any questions or comments.
The University of Michigan North Campus Long-Term Vision and LIDAR Dataset
I collected this dataset to evaluate long-term SLAM algorithms during my PhD work. It contains omni-directional imagery, 3D lidar and odometry data for 27 runs around campus over the course of a year.The dataset can be downloaded from: http://robots.engin.umich.edu/nclt
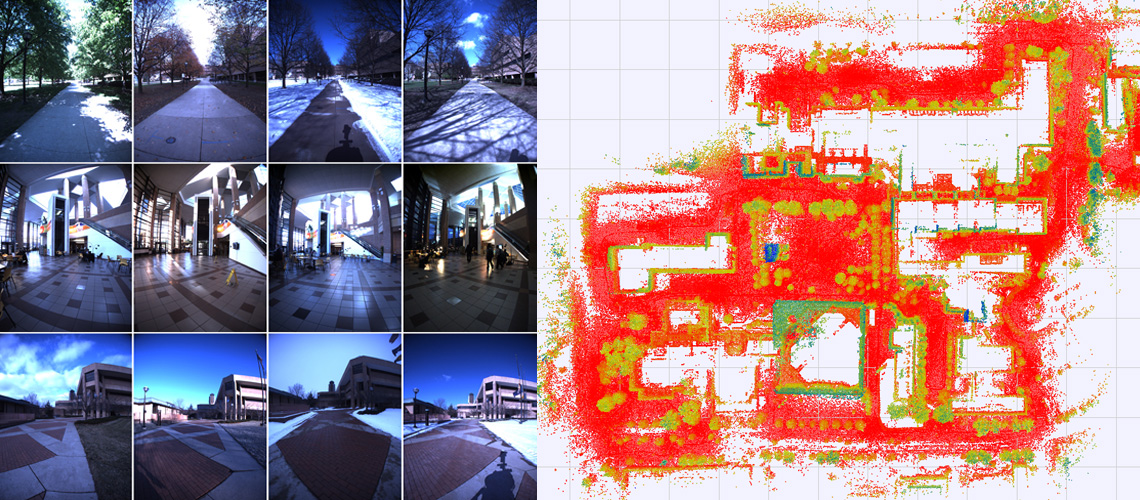
Past Projects
Next Generation Vehicle
PeRL is working on the "next generation" autonomous vehicle project — a collaboration with Edwin Olson's April Lab, Ford Motor Company and State Farm. Within the team, I worked on tools for building the prior maps and laser-based obstacle detection and tracking.Long-Term Mapping and Navigation
For my dissertation I worked on algorithms for long-term robotic mapping and navigation in dynamic environments.See the Publications and Code sections for more information.